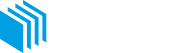
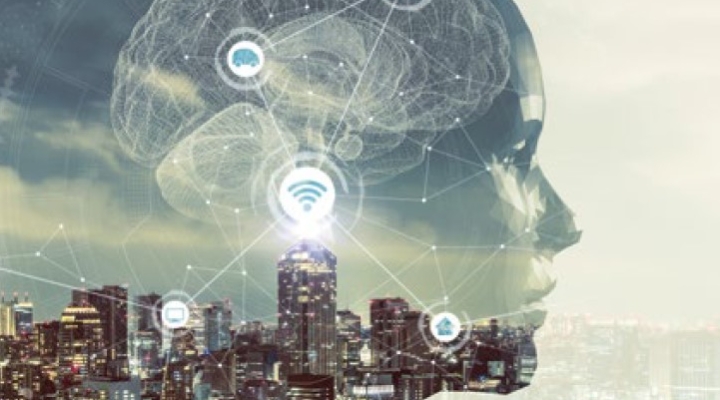
Artificial intelligence (AI) is attracting an enormous amount of attention in the media and public discourse. Well-publicised recent successes for AI have included self-driving cars and self-teaching board game champions.
Economists see AI as a nascent general purpose technology, capable of transforming working patterns in professional sectors, including law, in a way that some liken to the impact of the industrial revolution on manual labour.1 This disruption can bring great efficiencies, but also displace many human employees.2
Legal services are a major contributor to the UK economy, accounting in 2016 for 1.5% of domestic GVA and generating a trade surplus of £4bn.3 If implemented effectively, AI offers opportunities to improve legal services both for commercial parties and individuals. We are collaborating, along with an interdisciplinary team of academics at Oxford and a range of other private sector partners, on an ambitious programme of research into the potential application and limitations of AI to English law. The project, entitled Unlocking the Potential of AI for English Law,4 is funded by an award from UK Research and Innovation as part of its Next Generation Services investment programme.5 This in turn is one of a number of Industrial Strategy Challenge Funds, established to stimulate research partnerships between academia and the private sector in areas of importance to the UK economy.
The research project will investigate several of the (many) important questions raised for law and lawyers by the advent of AI. How is AI being used in legal services, and how does organisational structure and governance affect its implementation? What are the possibilities for the adoption of AI in dispute resolution?
Will lower costs facilitate access to justice? Will there be an impact on quality of provision that means the “justice” thereby provided is lacking in one or more important respects? What trade off, if any, should be adopted between cost and quality? What constitutional and other constraints are there on the use of AI in legal proceedings? Are there technological advances in the pipeline that may further push back the boundary between humans and machines in the future? How is AI adoption affecting job descriptions? What implications are there for the boundaries of professional knowledge, business models in legal services, and the education and training of lawyers and relevant technical specialists? In this article, we provide some background to these research questions along with some very preliminary insights from our work.
What is AI?
AI is not a new concept, the first usage generally being attributed to computer scientists John McCarthy and Myron Minsky in the mid-50s.6 The convention is to use the term in a functional sense, meaning that an artificial system functions as well as, or better, than a human. Clearly, machines can perform many tasks better than humans that do not involve intelligence, as opposed to strength or endurance. The “intelligence” qualifier therefore can usefully be understood as restricting the comparison to activities for which a human would use their brain – most obviously, processing and analysing information.
The classic assessment of whether a system functions as well as a human is the so-called “Turing test”, in which a human is asked to engage in conversation with messages sent through a mechanism that does not reveal whether the party on the other side is human or not. 7If a human participant cannot distinguish the communications of an artificial system from a human, then the test is passed by that system. To pass a Turing test without any constraints around the type of conversation that could be had, the machine would need to exhibit artificial general intelligence (AGI); that is, as good as human in every dimension of intelligence. Modern AI systems do not come anywhere near AGI. This is—according to experts—anywhere between a decade and two centuries away.8 Rather, the AI deployed today only has (super) human-level capability in respect of narrowly defined functions, such as image recognition, driving vehicles in straightforward surroundings, or the classification of documents.
Defining AI in this functional way means that no particular restrictions are put on the nature of the computing system used. Indeed, there have been at least three distinct technical approaches to AI since the birth of the field.9 The first, popular in the 1960s, involved logical rules: the idea was to develop a general-purpose system capable of deriving answers to problems through formal logical reasoning.10 This approach fell afoul of the problem that deterministic calculation of outcomes becomes exceedingly complex for even moderately challenging real-world problems.
A different tack was taken in the 1980s and 90s, with the advent of so-called “expert systems”.11 These were designed to give human users the benefit of expert answers to problems in an area of practice. The problems and answers – what computer scientists call “domain knowledge” – were characterised with the help of relevant human experts. These were then coded into a system designed to answer questions related to that particular body of knowledge. Expert systems in turn proved quite brittle, however. If the question asked by a user fell outside the system’s expertise, it could not give an answer. And moreover, the framing of questions had to be done in terms of the specific syntax of the system. If the user was unable to express themselves in terms the system could understand, then again it would fail. These created roadblocks to the roll-out of such systems.
Recent advances in AI rely primarily on machine learning (ML). This is an approach to computing in which the solution to an optimisation problem is not coded in advance, but is derived inductively by reference to data. The technique relies on applying computing power to very large amounts of data, the availability of which has blossomed in recent years.12 Progress since 2012 has largely been in a particular type of ML known as deep learning, which involves running multiple layers of representation of the data in series.13
The greatest practical successes with ML to date have been in the use of supervised learning techniques.14 This refers to a process that begins with a dataset that is classified or labelled by humans according to the dimension of interest, known as the training data. The system analyses this dataset and determines the best way to predict the relevant outcome variable (classified by the experts) by reference to the other available features of the data. The nature of the features, and the relationships between them, relevant for predicting the outcomes can be exceedingly complex: the power of ML lies in identifying the optimal mix of input variables. The trained model—that is, the algorithm with the set of parameters that optimised performance on the training dataset—is then put to work on a new test dataset, to see how effective it is at predicting outside the original training sample. These results must now be checked by human experts.
3. Applying AI to Law: Established Applications
One of our research questions is to understand the way in which AI is currently being applied in legal services. The foregoing account reveals two key constraints on the application of ML-based AI to legal contexts. First, the need for a large and relevantly labelled dataset for training the model. And second, the need for consistency between the training dataset and the data on which the trained model is to be used for predictive purposes.
Supervised learning techniques have been enormously effective in image recognition and language translation contexts, where vast quantities of pre-labelled data are available on the internet, and there is high consistency in the format of data. In many legal contexts, however,these conditions may prove more restrictive. Labelling a sufficiently large dataset of legal documents is costly, and the more varied the document types in question, the more difficult it may be to get good results. This means supervised learning techniques have fixed costs to implement, and their generalisability is constrained. They are consequently most useful in contexts where there is a very high volume of very similar material.
3.1 Technology-assisted review
The contexts in which ML-based techniques are now being actively applied in legal services are to identify relevant documents from amongst very large bodies of materials. In contentious matters, this is known as “technology-assisted review” (TAR). The growth of electronically stored information (ESI) means that there are enormous volumes of potentially relevant information for discovery/disclosure in a typical contentious matter. A large contentious matter can easily require review of hundreds of gigabytes of ESI per party.15 This has triggered a rapid rise in the costs of pretrial discovery relative to overall litigation costs, of which, in the US, over 70 per cent is estimated to be the costs of document review.16 This in turn has spurred uptake of ML applications in TAR (also referred to as “predictive coding”), which are able to deliver better results than human reviewers.17 TAR is also increasingly being used in large scale litigation in the UK.
To minimise the costs of training, practice has evolved whereby an iterative training process is deployed: an initial training set is used, the results of the test set are then checked and continuous review of outputs is performed to assist in improving the process as it develops.18 For TAR in relation to contentious matters, there is no scope for cross-matter application of a trained model, because the relevant identifying criteria are always highly idiosyncratic to the matter.
The use of TAR has been approved in various jurisdictions, including in particular the US; for example by Judge Peck in Monique Da Silva Moore v. Publicis Groupe & MSL Groupe,19 and in Rio Tinto v Vale.20 In the High Court in England, TAR was identified as a possible approach to the provision of e-disclosure in Goodale v The Ministry of Justice, 21 but was first specifically approved as a means of providing disclosure only in Pyrrho Investments Ltd v MWB Property Ltd.22 The use of TAR for large scale commercial litigation in the UK is, however, now well established. The pilot of a new disclosure regime in the Business and Property Courts that started on 1 January 2019 contains a sharper focus on the use of new technology. The Disclosure Review Document (DRD) requires parties to consider the use of TAR and states that, where parties have decided against it, particularly if the review universe is greater than 50,000 documents, they must set out reasoning as to why TAR should not be used.23There remain issues as to the application of TAR, including the selection of software and the appropriate method of selecting the test datasets and their checking.24 But such issues are small in comparison to the cost and considerable risk of human error when lawyers are tasked with reviewing vast quantities of electronically stored information.
3.2 AI in due diligence
Similarly, vast reams of documents must also be navigated in the context of transactional due diligence, again making it economic to apply supervised learning techniques. There may be greater scope for cross-matter application of a trained model as respects non-contentious matters, insofar as practitioners are looking for similar things amongst corporate documents in different transactions. The potential for cross-matter application is greatest where the documents are most standardised. Early successes
have been in contexts like real estate transactions. However, the applicability to other types of transactions is improving rapidly.
Practitioners using these tools rapidly come to learn that optimising performance involves a trade-off between various metrics.25 Perhaps the most intuitive is “precision”: the proportion of the documents selected by the trained model that are actually relevant to the matter in question.Low precision means that expensive human time must be spent reviewing documents that are not actually relevant – so-called “false positives”. However, simply designing a system to maximise precision is not the answer. Another relevant metric is “recall”: to what extent does the system capture relevant documents from the corpus as a whole. Low recall rates mean that the system generates a high number of “false negatives”— that is, it treats documents as not relevant when they actually are. Focusing simply on maximising precision can often result in a system that appears to be delivering good results (“it only sends me relevant stuff”) but in fact is missing out large amounts of relevant material in the process. Determining recall rates requires review not just of the documents selected by the system, but of samples of the underlying dataset.
As the breadth of applicability of supervised learning solutions depends on consistency between documents, there are clearly opportunities for increasing their utilisation by increasing consistency in particular types of contract.
4. Emerging Applications
A fast-growing application, and one that may be of particular concern to lawyers worried about being replaced by robots, is the use of technology to predict case outcomes. Already available are tools that mine and aggregate data from prior disputes to give parties information about the prior record of particular judges and lawyers. These data can then be fed into a supervised learning model to predict outcomes. Early work has produced results achieving in excess of 70 per cent accuracy in predicting success in relation to disputes in the ECHR and US Supreme Court, respectively.26 However, the very heterogeneous nature of disputes means that there is considerable variation by matter type, and early commercially-available versions of this type of technology are focusing on particular dispute types to achieve better accuracy – e.g. patent, antitrust, etc.27
The publicly-available record often only contains the outcome of fact-determination exercises; that is, it does not contain all the evidence submitted by the parties but just the findings of fact made by the decision-maker or statements of agreed facts. This means that early progress is likely to be made in predicting outcomes given particular facts. This is a parallel process to the one in which a lawyer might assess the likely outcome by applying the law to the facts. With a supervised learning approach, the AI is not in fact applying the law. Rather, it is modelling statistical relationships between the language in prior disputes to determine the likely outcome in this matter, given the nature of the facts. However, from the standpoint of commercial parties, predicting the likely outcome with a sufficient level of accuracy is often likely to be enough. A prediction permits parties to determine an appropriate settlement value, and avoid the costs of litigation. Of course, the heterogeneity of dispute types mean that there will be likely to be much variation in predictions based even on the application of supervised learning to prior disputes; that variation will impede its utility for settlement purposes.
The application of supervised learning models to precedent data raises a host of important questions, both practical and normative. From a practical standpoint, could commercial parties, commercially rather than simply using supervised learning for estimating outcomes, embed an appropriately trained model within an arbitral or other dispute resolution mechanism? Such a mechanism would provide a cheap means of resolving disputes that could offer tolerable congruity with the outcomes of human decision-making, particularly for parties frequently involved in disputes, across which individual errors could average out. Moreover, lowering costs automation can also facilitate equality of arms between the parties. The level of accuracy needed to be “tolerable” for commercial parties, interested only in financial consequences of disputes, is likely to be lower than for individuals concerned with issues touching on fundamental rights. This raises challenging questions concerning the mechanisms by which consent may be given to channel a dispute to an automated resolution procedure, and the circumstances under which such a process might be subject to (human) judicial review.29 And under what circumstances—if any—could the output of such analytics have normative significance in a dispute before a human adjudicator?
A significant limitation in the use of ML-based AI in legal advice is the lack of transparency concerning factors relevant to the prediction. ML can give an expected outcome—and perhaps even a quantum—but generally cannot provide any sort of readily-interpretable explanation behind this. This in turn poses further questions, well-put by Richard Susskind: to what extent do parties actually need legal advice? 30 A “front end” for a system based on ML could be framed in terms of lay questions, to which the user could provide answers, and an outcome is automated. Simple matters such as conveyancing, lease agreements and wills can readily be automated; personal injury could be turned into a liability estimation mechanism, and so forth.
A risk inherent in the application of ML to existing datasets of human practice is that the data may reflect some element of bias in prior decisions against persons in respect of (now) protected characteristics.31 Given changing attitudes—and law—over time, it seems plausible that such bias is more likely to be present for older decisions. ML applications coded on such data may simply replicate this bias. Because ML cannot explain how results are achieved, it is not possible simply to examine the process of reasoning.32 Instead, it is necessary to explore other mechanisms for ensuring that the decision is free from discrimination.33
A further step, still, would be the application of such technology to the determination of disputes which, at present, are resolved by a judge in court. Could this provide a possible solution to issues raised by the shortage of legal aid or by the inability of SMEs to fund litigation? Attempting to apply existing technology in this way would, however, quickly run into obvious and potentially fundamental obstacles, including where there is a requirement for public hearings or for reasons to be given for any decision. In Flannery v Halifax Estate Agencies Ltd,34 Henry LJ said that the duty of a judge to give reasons is a function of dueprocess, and therefore of justice.35 Its rationale has two principal aspects. The first is that fairness requires that the parties, and especially the losing party, should be left in no doubt why they have won or lost. This is especially so since without reasons the losing party will not know whether the court has misdirected itself, and thus whether he may have an available appeal on the substance of the case. The second is that a requirement to give reasons concentrates the mind; if it is fulfilled, the resulting decision is much more likely to be soundly based on the evidence than if it is not. However, one may question how far this rationale extends if both parties have agreed to a judgment being given without reasons.
For the moment, at least, it looks unlikely that AI is going to be able to fulfil requirements such as these. A more difficult question may, however, be how one deals with a situation in which, if AI is not to be permitted to be used to adjudicate disputes, the individual will be unable to litigate and thus unable, in any event, to enjoy the benefit of a public hearing and a decision, reasoned or otherwise, on his or her claim. Perhaps a technological solution may emerge. In the future, emerging semantic systems approaches in AI research, which seek to combining the structured reasoning of the expert systems approach with the inductive engagement with data of ML, may offer a way to move beyond the “black box” character of current applications. This is an exciting avenue for future research at the intersection of computer science and law.36
Conclusion
The application of AI to law raises many interesting and challenging questions, a number of which our research project will explore over the course of the next two years. While the hyperbole associated with AI has surely engendered unrealistic expectations, only a fraction of these need to be met for the changes to be profound.
1See e.g. E Brynjolfsson, D Rock, and C Syverson, ‘Artificial Intelligence and the Modern Productivity Paradox: A Clash of Expectations and Statistics’ and M Trajtenberg, ‘AI as the Next GPT: A Political-Economy Perspective’, in AK Agrawal, J Gans and A Goldfarb (eds.), The Economics of Artificial Intelligence: An Agenda (Chicago: University of Chicago Press, forthcoming 2019).
2See e.g. J Furman and R Seamans, ‘AI and the Economy’ in J Lerner and S Stern, Innovation Policy and the Economy 2018, Vol 19 (Chicago: University of Chicago Press, forthcoming 2019).
3TheCityUK, UK Legal Services 2017: Legal Excellence, Internationally Renowned (London: TheCityUK, 2017).
4 See https://www.law.ox.ac.uk/researchand-subject-groups/unlocking potentialartificial-intelligenceenglish-law/workpackages.
5https://www.ukri.org/innovation/industrial-strategychallenge-fund/nextgeneration-services/.
6 J McCarthy, ML Minsky, N Rochester and CE Shannon, A Proposal for the Dartmouth Summer Research Project on Artificial Intelligence (1955) (proposing a “2 month, 10 man study of artificial intelligence”, organised around “the conjecture that every aspect of learning or any other feature of intelligence can in principle be so precisely described that a machine can be made to simulate it.”).
7AM Turing, ‘Computing Machinery and Intelligence’ (1950) 49 Mind 433, 434. A test so formulated “has the advantage of drawing a fairly sharp line between the physical
and the intellectual capacities of a man.” (ibid).
8Martin Ford, Architects of Intelligence (Birmingham: Pakt Publishing,2018), 528-9.
9See S Russell and P Norvig, Artificial Intelligence: A Modern Approach, 3rd ed. (Upper Saddle River, NJ: Pearson, 2010), 16-28.
10See generally, J Minker, ‘Introduction to Logic-Based Artificial Intelligence’, in J Minker (ed.), Logic-Based Artificial Intelligence (Dordrecht: Kluwer, 2000), 3.
11See e.g., P Harmon and D King, Expert Systems: Artificial Intelligence in Business (New York: Wiley, 1985). For a classic account of their application to law, see R Susskind, Expert Systems in Law: A Jurisprudential Enquiry (Oxford: Clarendon Press, 1987).
12 See A Halevy, P Norvig and F Pereira, ‘The Unreasonable Effectiveness of Data’ (2009) IEEE Intelligent Systems 8.
13See e.g., F Chollet, Deep Learning with Python (Shelter Island, NY: Manning, 2018), 8-11.
14See Ford,supra n 8, 186.
15NM Pace and L Zakaras, Where the Money Goes: Understanding Litigant Expenditures for Producing Electronic Discovery (Santa Monica, CA: RAND Corp, 2012), 29-31.
16Ibid, 42.
17Grossman and GV Cormack,‘Technology-Assisted Review in e-Discovery can be More Effective and More Efficient than Exhaustive Manual Review’ (2011) 17 Richmond Journal of Law & Technology 11; Pace and Zakaras, supra n 15, 65-66.
18See GV Cormack and MR Grossman, ‘Evaluation of Machine-Learning Protocols for Technology-Assisted Review in Electronic Discovery’ (2014) Proceedings of the 37th international ACM SIGIR conference on Research & development in information retrieval 153.
19868 F.Supp.2d 137 (SDNY, 2012).
20306 F.R.D. 125 (SDNY, 2015).
21[2009] EWHC 3834(QB).
22[2016] EWHC 256(Ch).
23Practice Direction 51U – Disclosure Pilot for the Business and Property Courts, Appendix 2: Disclosure Review Document, 20.
24See e.g., Rio Tinto, supra n 20, at 128; see also SH Kitzer, ‘Garbage In, Garbage Out: Is Seed Set Disclosure a Necessary Check on Technology-Assisted Review and Should Courts Require Disclosure?’ [2018] University of Illinois Journal of Law, Technology and Policy 197.
25See e.g. Pace and Zakaras, supra n 15, 117-9; Rio Tinto, supra n 20, at 130.
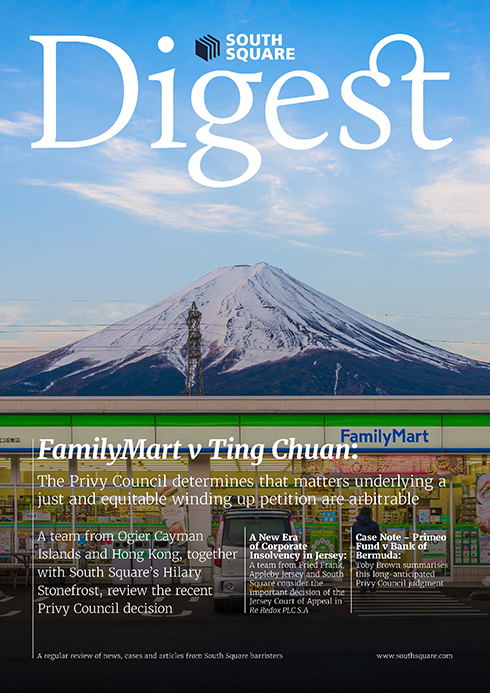